Dataset for Sim2Real Meta-Learning-based Training for mmWave Beam Selection in V2X Networks
Download our dataset and code:
Please use below link to download the datasets:Please use below link to our github repository:
https://github.com/genesys-neu/FLASH-MAML
Description:
The dataset used in our SMART framework consists of two main components. The real-world e-FLASH dataset is a multimodal collection featuring synchronized LiDAR, camera, and GPS data captured in diverse mmWave V2X scenarios—including LOS, NLOS with pedestrian, static car, and moving car obstacles—totaling over 10,853 samples (∼22 GB processed data). The synthetic S-FLASH dataset is a high-fidelity digital twin recreation of the e-FLASH environment along a two-lane urban road. It is generated using open-source tools (Blender for image synthesis and Blensor for LiDAR simulation) combined with Wireless InSite for detailed ray-tracing, resulting in 26,600 samples (∼90 GB processed data). Together, these datasets enable robust training and evaluation for mmWave beam selection while facilitating effective synthetic-to-real domain adaptation via meta-learning.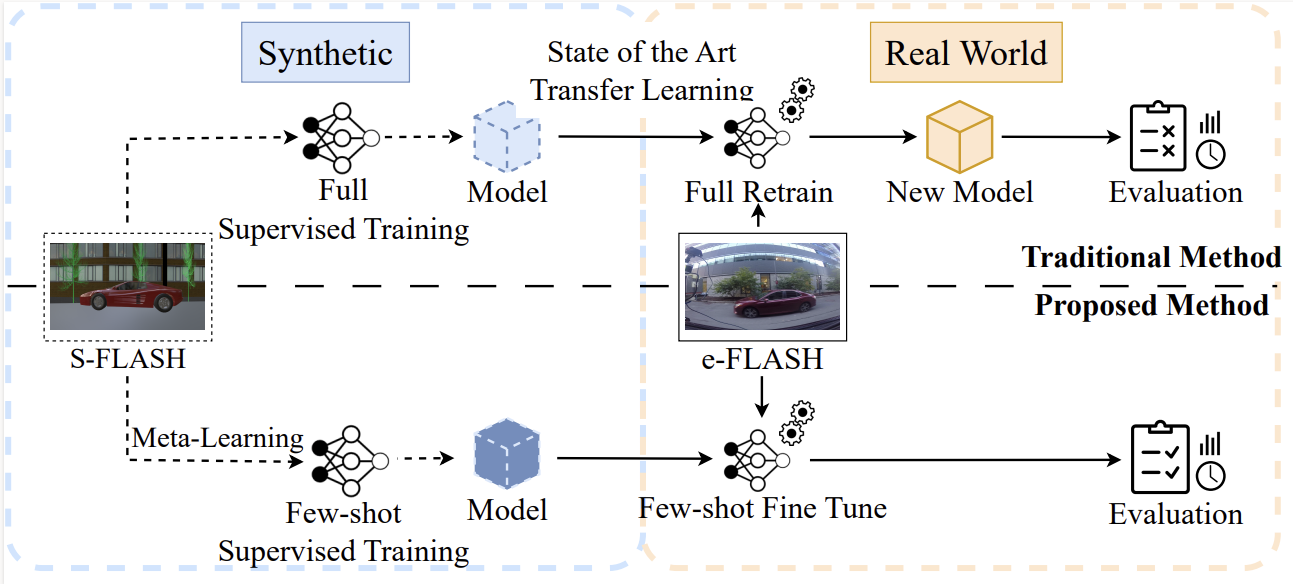
Fig. 1: The proposed methodology based on meta-learning out-performs the traditional transfer-learning-based method in terms of accuracy, the amount of data used for training, and end-to-end computation time.
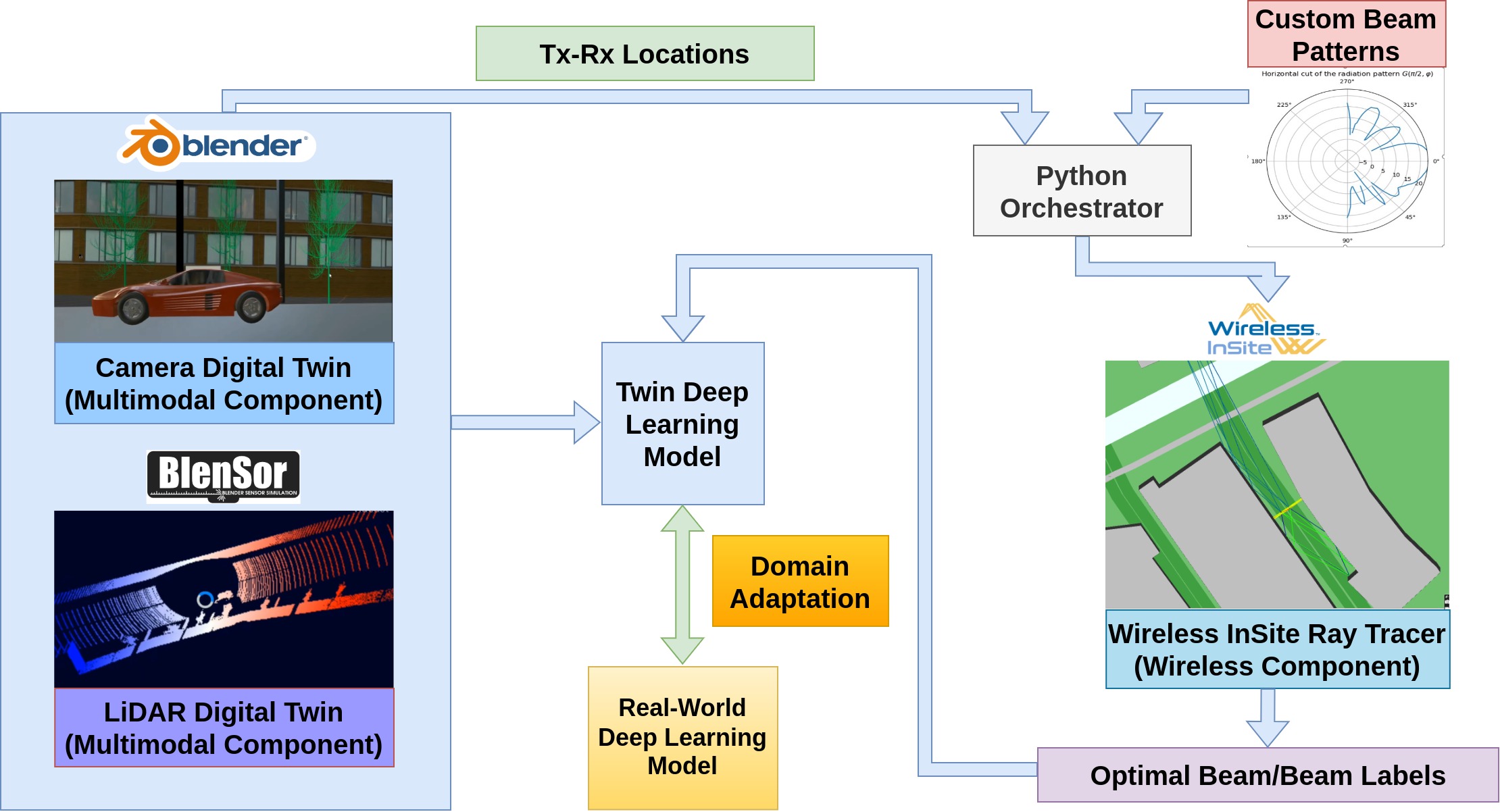
Fig. 2: Orchestration process between the DT components and the DL models within the SMART framework to collect and process the multimodal sensor data and ground truth beam labels.
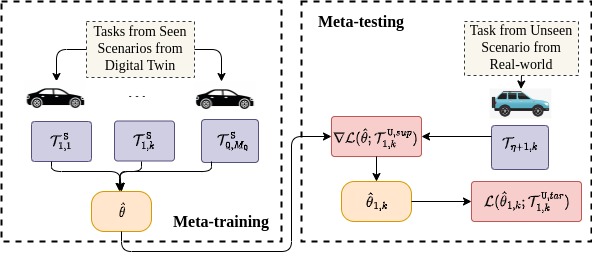
Fig. 3: Proposed MAML-based framework for adapting to unseen scenarios for beam selection.
Experimental Setup
For real-world data collection in our SMART framework, we employ a Lincoln Mkz Hybrid autonomous car outfitted with advanced sensors and communication equipment. The vehicle is equipped with a GPS system and a state-of-the-art Ouster OS1-64 channel LiDAR that provides a 360-degree panoramic view. These sensors are integrated with an onboard computer running the Robot Operating System (ROS) for precise data logging, storage, and synchronization. To support mmWave connectivity experiments, we utilize Talon TP-Link AD7200 tri-band routers—equipped with Qualcomm QCA 9500 Wi-Fi chips—that operate in the 60 GHz band. One router is mounted on the vehicle’s roof to serve as the receiver, while three additional routers, positioned at 20-meter intervals along a straight line, function as roadside units (RSUs). This comprehensive setup facilitates robust evaluation of mmWave beam selection and V2X connectivity under realistic conditions.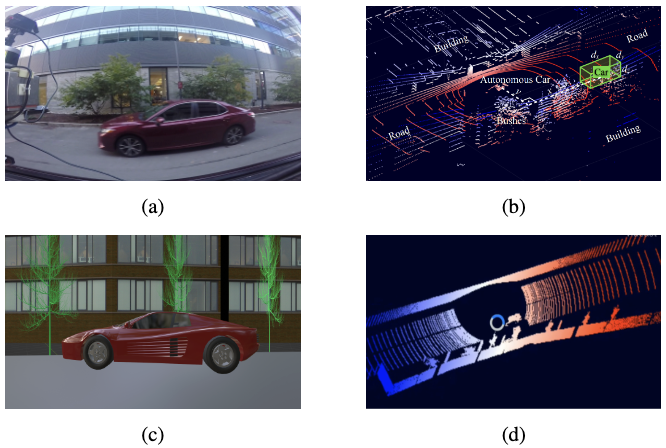
Fig. 4: Samples taken from the e-FLASH and S-FLASH dataset with a camera image and LiDAR pointcloud side-view pairing from the real world (a), (b) and a camera image and LiDAR pointcloud front-view pairing from the synthetic environment (c), (d), respectively. The images for the LiDAR captures were visualized in MATLAB LiDAR Toolbox.
Dataset Description:
We collect the SMART dataset replicating the real-world vehicular network scenarios, in: A) Category 1: LOS passing, B) Category 2: NLOS pedestrian, C) Category 3: NLOS static car, D) Category 4: NLOS moving car.Category | Featuring | Scenario | No. of Episodes | No. of Samples |
---|---|---|---|---|
1 | - | LOS | 10 | 1900 |
2 | Pedestrian | NLOS | 30 | 5700 |
3 | Static Car | NLOS | 10 | 1900 |
4 | Moving Car | NLOS | 20 | 3800 |