Dataset for leveraging Co-Operative Perception using LiDAR for Handoffs between Road Side Units
Download our dataset and code:
Please use below link to download the datasets:Captured GPS, LiDAR and wireless connectivity data from a V2X environment under 4 varying categories
Please use below link to our github repository:
https://github.com/genesys-neu/COPILOT
This dataset was used for the paper "COPILOT: Cooperative Perception using Lidar for Handoffs between Road Side Units" to be published in IEEE INFOCOM 2024. Please use this link to download the paper. Any use of this dataset which results in an academic publication or other publication which includes a bibliography should include a citation to our paper. Here is the reference for the work:
Conference version: PDF
S. Pradhan, D. Roy, B. Salehi, K. Chowdhury, "COPILOT: Cooperative Perception using Lidar for Handoffs between Road Side Units,” IEEE INFOCOM, Vancouver, Canada, May 2024.
Description:
COPILOT presents a ML-based ap- proach that allows vehicles requiring ubiquitous high bandwidth connectivity to identify the most suitable road side units (RSUs) through proactive handoffs. By cooperatively exchanging the data obtained from local 3D Lidar point clouds within adjacent vehicles and with coarse knowledge of their relative positions, COPILOT identifies transient blockages to all candidate RSUs along the path under study. COPILOT proposes three modules that operate in an inter-connected manner: (i) As an alternative to sending raw Lidar point clouds, it extracts and transmits low-dimensional intermediate features to lower the overhead of inter-vehicle messaging; (ii) It utilizes an attention-mechanism to place greater emphasis on data collected from specific vehicles, as opposed to nearest neighbor and distance-based selection schemes, and (iii) it experimentally validates the outcomes using an outdoor testbed composed of an autonomous car and Talon AD7200 60GHz routers emulating the RSUs, accompanied by the public release of the datasets. Results reveal COPILOT yields upto 69.8% and 20.42% improvement in latency and throughput compared to traditional reactive handoffs for mmWave networks, respectively.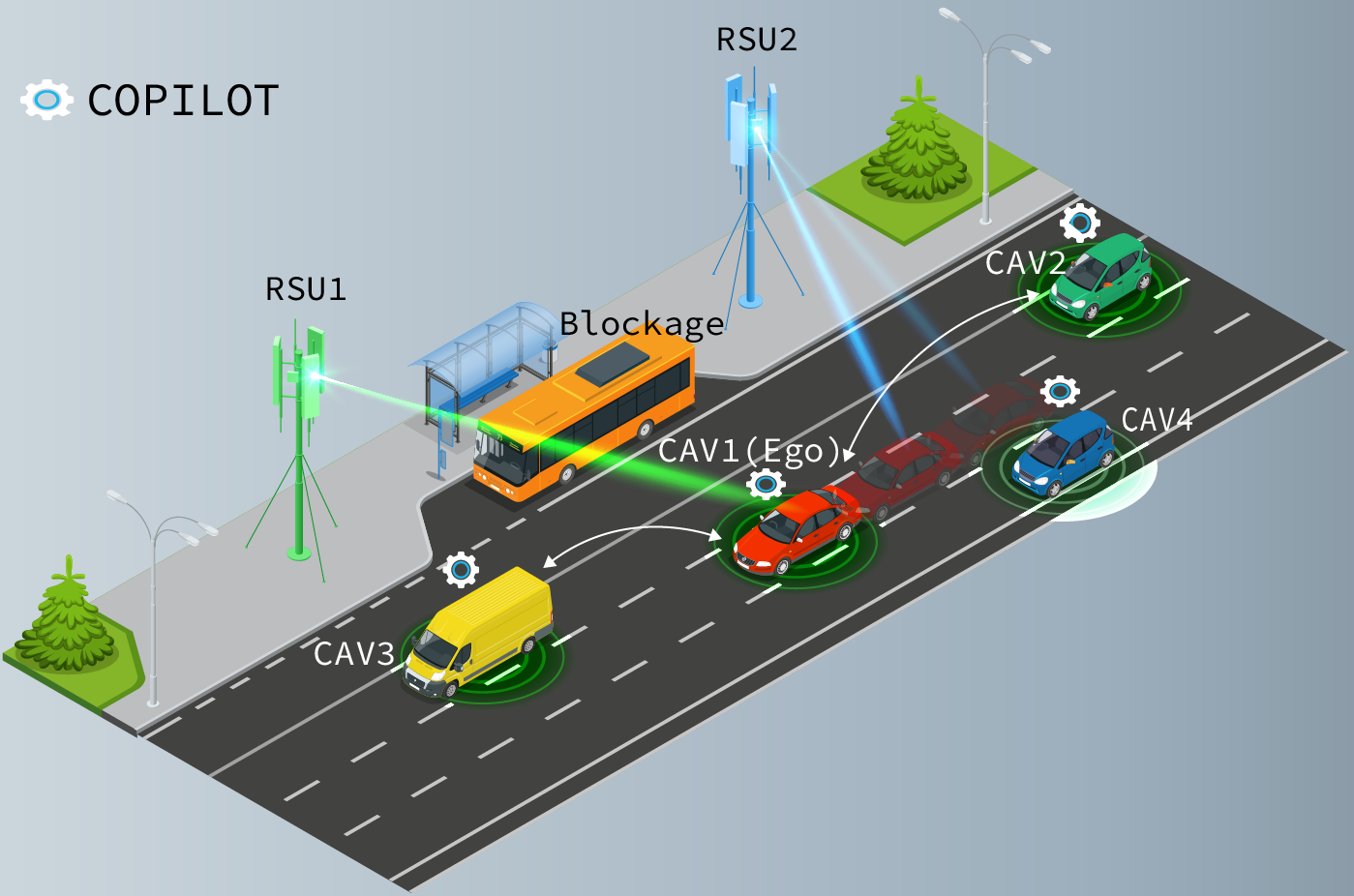
Fig. 1: V2X mmWave scenario of interest and deployment of COPILOT framework to enable proactive handoff
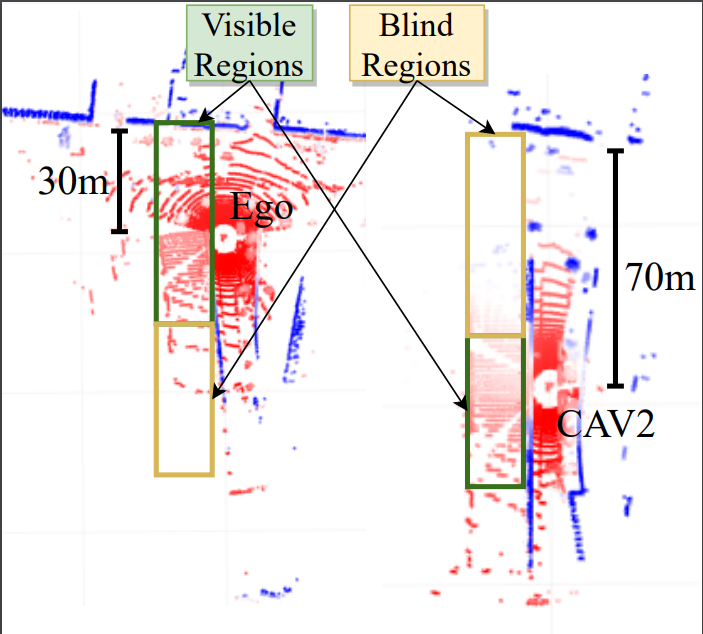
Fig. 2: Motivation for Co-operative Perception using LiDAR point clouds to compensate for blind regions in a capture
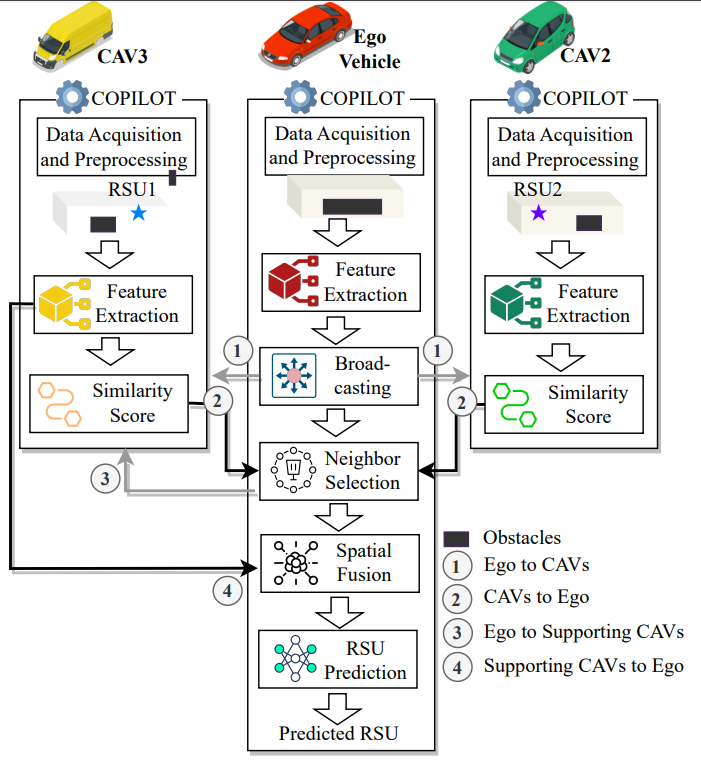
Fig. 3: COPILOT System Architecture showcasing 'Ego Vehicle' predicting the optimal RSU using extracted features from strategically selected vehicles CAV2 and CAV3
Experimental Setup
We use a Lincoln Mkz Hybrid autonomous car equipped with a GPS system and a state-of- the-art Ouster OS1-64 channel Lidar that cpatures a panoramic 360-degree field of view. These sensors are integrated with an on-board computer running robot operating system (ROS) for logging location, data storage and synchronization. We utilize Talon TP-Link AD7200 tri-band routers, equipped with Qualcomm QCA 9500 Wi-Fi chips, to establish connectivity in the 60 GHz mmWave frequency band. One of these routers is mounted on the roof of our autonomous vehicle to serve as a receiver, while the remaining three function as RSUs, separated by 20m along a straight line.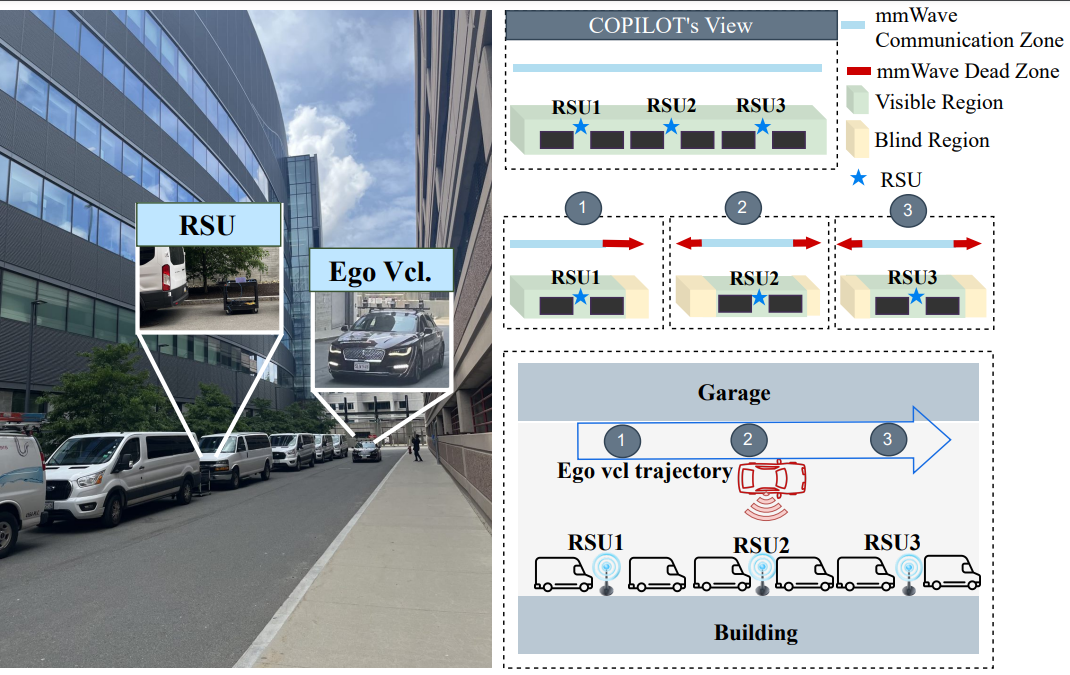
Fig. 4: Real-world data collection setup for COPILOT (left) showing a partial view of the road with one RSU and the ego vehicle.
Dataset Description:
We collect the COPILOT dataset repli- cating the real-world vehicular network scenarios, categorized in: (a) Line of Sight (LOS), (b) Non-Line of Sight (NLOS) with a pedestrian in front of the RSU, (c) NLOS surrounded by vehicles around the RSU, (d) NLOS surrounded by vehicles and a pedestrian walking around the RSU.Category | Blockage | Scenario | No. of Episodes | No. of Samples |
---|---|---|---|---|
1 | No Obstacle | LOS | 10 | 3066 |
2 | Pedestrian | Static | 10 | 2826 |
3 | Vehicle | Static | 10 | 2384 |
4 | Static Vehicle and Pedestrian | Left to Right | 5 | 1425 |
Right to Left | 5 | 1367 |